The Potential Of Artificial Intelligence In Healthcare
Artificial intelligence (AI) will be used more and more in the healthcare industry as a result of the complexity and growth of data in the sector. There are several different AI technologies in use today by payers, healthcare providers, and life sciences companies. Recommendations for diagnosis and treatment, patient involvement and adherence, and administrative chores are the key application categories. Although there are many situations in which AI can execute healthcare services just as well as or better than humans, implementation issues will keep the jobs of healthcare professionals from becoming extensively automated for a substantial amount of time. The use of AI in healthcare and ethical concerns are still controversial.

Overview
AI and similar technologies are becoming more and more common in business and society, and they are starting to be used in healthcare. Several facets of patient care could be changed by this technology, as well as internal administrative procedures among payers, providers, and pharmaceutical organizations.
Several studies have already shown that AI is capable of doing important healthcare jobs including disease diagnosis and even better than humans. Today, algorithms already surpass radiologists in identifying cancerous tumors and advising researchers on how to create cohorts for expensive clinical trials. Nonetheless, we think it will be a long time before AI completely replaces humans in large medical process domains for a variety of reasons. In this article, we discuss both the potential of automation in healthcare and some of the obstacles that stand in the way of its quick adoption.
Types of AI in Healthcare
Many different technologies make up artificial intelligence. Although the majority of these technologies are immediately applicable to the healthcare industry, the precise procedures and jobs they assist differ greatly. Below are definitions and descriptions of some specific AI technologies that are very significant to healthcare.
Machine learning

Machine learning is a statistical method for ‘learning’ through ‘teaching’ models with data and fitting models to data. One of the most prevalent types of AI is machine learning; in a 2018 Deloitte poll of 1,100 US managers whose organizations were already exploring AI, 63% of the businesses surveyed were using machine learning (Thomas Davenport & Ravi Kalakota, 2019). There are numerous variations of this broad strategy, which forms the basis of many AI methodologies.
Precision medicine, which determines which treatment procedures are likely to be effective on a patient based on a variety of patient traits and the context of the therapy, is the most popular application of traditional machine learning in the healthcare industry. The vast majority of machine learning and precision medicine applications ask for supervised learning, which calls for a training dataset for which the end variable (such as the onset of disease) is known.
Natural language processing
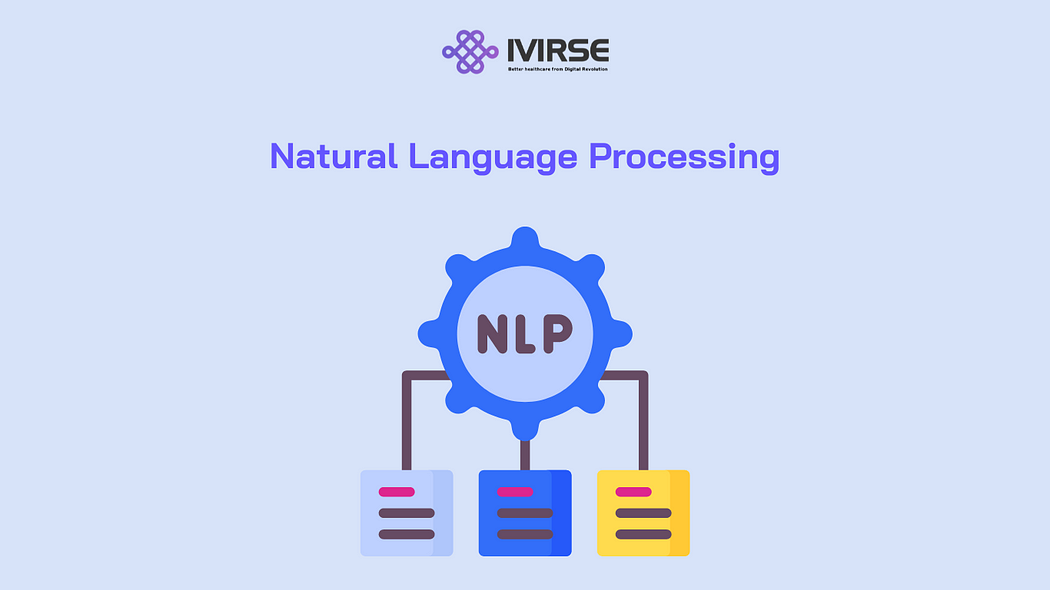
Since the 1950s, AI researchers have been trying to understand how human language works. Natural Language Processing (NLP) is a field that comprises language-related applications like speech recognition, text analysis, translation, and others. The two main methods are statistical NLP and semantic NLP. Statistical NLP is based on machine learning, specifically deep learning neural networks, and has helped to improve recognition accuracy recently. To learn it, you need a sizable “corpus” or body of language. NLP systems are able to conduct conversational AI, create reports (for example, on radiological examinations), analyze unstructured clinical notes on patients, and record patient interactions.
Rule-based expert systems
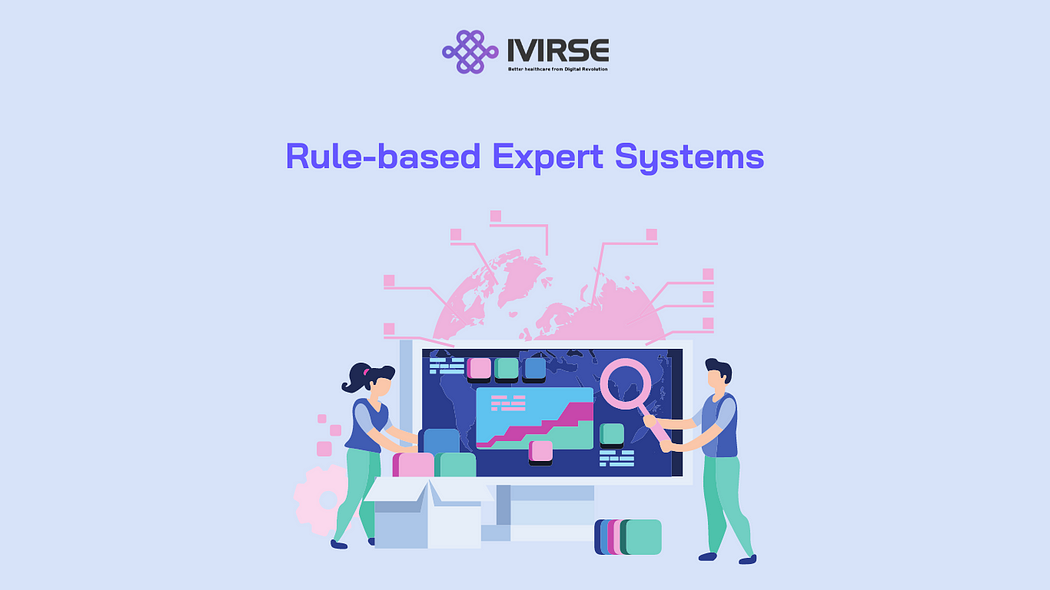
Throughout the 1980s and succeeding decades, expert systems built on databases of “if-then” rules dominated the field of artificial intelligence. For the past two decades, they have been extensively used in the healthcare industry for “clinical decision support” purposes, and they are still frequently used today (Vial, Stirling & Field, 2018). Today, a lot of suppliers of electronic health records (EHRs) provide a set of guidelines with their systems.
A set of rules in a certain knowledge domain must be built by human experts and knowledge engineers for expert systems. They are simple to understand and operate well up to a point. Unfortunately, they frequently fail when there are a lot of rules (typically over a few thousand) and when the rules start to conflict with one another. Also, it can be challenging and time-consuming to update the rules if the knowledge domain does. More approaches based on data and machine learning algorithms are gradually replacing them in the healthcare industry.
Physical robots

Given that more than 200,000 industrial robots are installed each year worldwide, physical robots are at this point well-known. In settings like factories and warehouses, they carry out predetermined activities including lifting, moving, welding, or assembling goods, as well as transporting supplies in hospitals. More recently, robots have improved their ability to work cooperatively with people and are simpler to teach by having them perform a desired activity. Also, they are developing greater intelligence as additional AI capabilities are integrated into their “brains” (really their operating systems). It would appear reasonable that over time, physical robots would include the same advancements in intelligence that we have seen in other branches of artificial intelligence.
Surgical robots give surgeons “superpowers,” enhancing their vision, and capacity to make precise, minimally invasive incisions, close wounds, and other surgical procedures (Davenport & Glaser, 2002). They were first approved in the USA in 2000. Yet, significant choices are still made by human surgeons. Gynecologic surgery, prostate surgery, and head and neck surgery are among the common surgical procedures performed with robotic surgery.
Robotic process automation

This technology executes organized digital administration tasks — those involving information systems — as if they were being carried out by a human user who was following a set of instructions or guidelines. They are less expensive, simpler to program, and more transparent than other types of AI. Robotic process automation (RPA) primarily uses server-based software rather than actual robots. To behave as a semi-intelligent user of the information systems, it depends on a combination of workflow, business rules, and ‘presentation layer’ integration. They are employed in the healthcare industry for routine duties like billing, prior authorization, and patient record updates. They can be used to extract data from, say, faxed photographs and feed it into transactional systems when paired with other technologies like image recognition (Hussain, Malik, Halim & Ali, 2014).
Although we have only discussed these technologies individually, they are progressively being combined and integrated. For example, robots are acquiring AI-based “brains,” and RPA and image recognition are being combined. Perhaps these technologies will become so intertwined in the future that composite solutions will become more possible or practical.
The future of AI in healthcare
AI will have a significant impact on future healthcare services. It is the main capability underlying the development of precision medicine, which is universally acknowledged to be a critically needed improvement in healthcare. It takes the form of machine learning. Although early attempts at making recommendations for diagnosis and therapy have been difficult, it is anticipated that AI will eventually become proficient in that field as well. It appears likely that the majority of radiology and pathology images will eventually be reviewed by a computer given the rapid advancements in AI for imaging analysis. It will become more common to use speech and text recognition for purposes like patient communication and clinical note transcription.
Not determining whether the technologies will be capable enough to be beneficial, but rather guaranteeing their acceptance in routine clinical practice, is the biggest hurdle for AI in various healthcare sectors. In order for AI systems to be widely adopted, they need to be endorsed by regulatory bodies, integrated with EHR systems, sufficiently standardized so that similar products function similarly, taught to clinicians, paid for by public or private payer organizations, and improved over time in the field.
References
Davenport, T. H., & Glaser, J. (2002). Just-in-time delivery comes to knowledge management. Harvard business review, 80(7), 107–11.
Hussain, A., Malik, A., Halim, M. U., & Ali, A. M. (2014). The use of robotics in surgery: a review. International journal of clinical practice, 68(11), 1376–1382.
Vial, A., Stirling, D., Field, M., Ros, M., Ritz, C., Carolan, M., … & Miller, A. A. (2018). The role of deep learning and radiomic feature extraction in cancer-specific predictive modeling: a review. Transl Cancer Res, 7(3), 803–816.
Comment
Latest news
2025 Healthcare Breakthroughs: Trends to Watch!
Most viewed
Mind Matters: Prioritizing Mental Health with OOMY
In today’s fast-paced world, mental health is often overlooked. OOMY is here to change that by putting your well-being first. With its suite of mental health features, this app acts as your digital companion, guiding you through life’s ups and downs.
Unveil Your Inner Voice: OOMY’s Random Tarot Messages
Do you ever feel like the universe is trying to tell you something? OOMY’s Random Tarot feature brings a touch of magic to your everyday life. Whether you’re seeking guidance, inspiration, or just a moment of reflection, these cards offer a doorway to self-discovery.
Walk Your Way to Wellness: Discover Happy Hike on OOMY
Winter is here, and staying active can be a challenge. With OOMY’s Happy Hike feature, walking becomes more than just a physical activity – it’s a holistic experience that connects you to nature and your inner self. Whether you’re strolling through a park, jogging along a trail, or simply taking steps around your neighborhood, every movement matters.
Embrace Winter Wellness with OOMY's Meditation Feature
Winter mornings can be tough. The cold weather tempts us to stay in bed a little longer, leaving us feeling sluggish and uninspired. However, starting your day with morning meditation can change your entire outlook. Let’s explore why and how OOMY’s meditation feature can help you embrace the chill while prioritizing your mental and physical health.